(And an Introduction to the Machine Learning Lifecycle)
Many organisations struggle with the successful adoption of Machine Learning, which can lead to significant expenditure on projects that return little value.
Failure in this sense can also have wider-reaching ramifications for the business - such as creating difficulties to secure budget or internal buy-in for future Machine Learning projects that could make a significant positive impact on areas of the organisation.
There are a few reasons why this typically occurs, as we have touched on in recent blogs. In this post we’ll outline five key considerations for those considering the adoption of Machine Learning, and how they can confidently get off on the right foot.
Typical Pitfalls and How to Overcome Them
In our experience, the single largest reason for failure of ML projects is that they are technology-led. Specifically, this happens when technology teams within the organisation decide to look at the art of the possible, and build proofs of concept without any substantial level of business user engagement.
When the time comes to deliver these into production, the lack of engagement across the business means that the PoC may have missed the point, it will most likely not have been budgeted for, and it doesn't necessarily capture all the nuances of the particular problem (if it is even a real-world challenge) that the business would expect.
One of the recurring topics in this blog series has been around the benefits of taking down fences between teams in delivery, which can have fruitful business-wide implications - but in this context, bridging the gap between Machine Learning and Software Delivery is especially critical.
Just as important is extending the same approach and converging your ML/IT team and your business team. The best Machine Learning units have a small, but deeply expert, number of resources to reflect the business domain.
The Recommendations
Some of our key recommendations to clients to ensure successful Machine Learning delivery include:
- Involve stakeholders from all three domains (Machine Learning, IT and Business) from the start. IT’s involvement may be more limited initially (depending on your analytics platform maturity, and data maturity), but it is important the project appears on their radar from the outset.
- Never discuss techniques - techniques are a means to a solution. In the same way that IT is unlikely to get into the minutiae of Java versus C# when working with the business team, a successful Machine Learning conversation might only skim over the techniques at a very high level (such as prediction, segmentation, computer vision, and natural language processing). There will inevitably be a number of stakeholders who are very interested in the details, and that is a useful discussion to have at a later time in a more casual setting, but it is unlikely to be conducive to the outcome.
- Focus on the business strategy and current challenges. Understanding the current strategy allows you to solve problems that align with areas of business concern more likely to receive funding. Grasping existing pain points also allows you to help define quick return on investment criteria. Deep-dive into these topics with examples and case studies to help understand where a Machine Learning-based solution may provide help, and use this to triage each of the business problems by relevance and viability.
- Follow the money - inevitably in the chain of cashflow for an organisation, predictions become critical. For a retailer, that might mean predicting what someone is likely to buy, or what volume of inventory is needed to satisfy likely demand. For a bank, it might be predicting volume of trade for a particular product and interest rate. Following the money makes it easier to find where an application of Machine Learning is likely to give substantial return on investment.
- Start medium, then go large. Avoiding the most sensitive challenges in an organisation is critical for nascent ML teams - they can be a political minefield of vested interests and differing opinions, as well as extremely rigorous change management. Disruption in quality of predictions in the most critical products will be very visible, whereas working on things that are slightly less important for the organisation gives the opportunity to deliver value as well as the chance to get it wrong (which is also extremely important), and to mature the Machine Learning team.
The ML Lifecycle
Appsbroker, through our experience of delivering Machine Learning (to production, not just Proof of Concepts!), have built a modified ML Lifecycle to articulate the different stages of delivery. For technical data scientists, this essentially represents an organisationally-aware extension of the CRISP-DM approach.
We will build more on this diagram in a future instalment, so stay tuned!
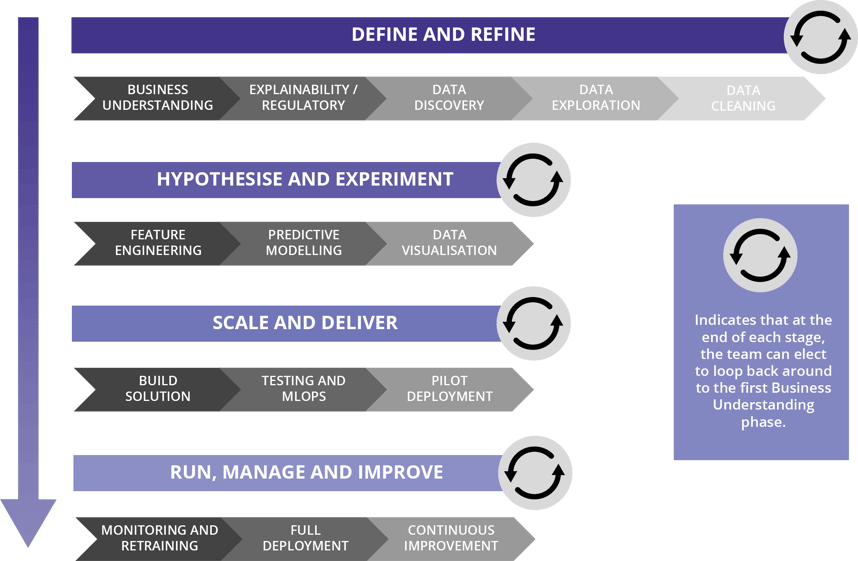
Don't forget to subscribe below to kept up-to-date with the latest on data & machine learning!
The Analytics Revolution blog series: